Google says its AI supercomputer is faster, greener than Nvidia A100 chip
- Google’s own custom chip, called the Tensor Processing Unit, is used for more than 90 per cent of the company’s work on AI training
- Google published a paper detailing how it has strung more than 4,000 of the chips together into a supercomputer using its own optical switches
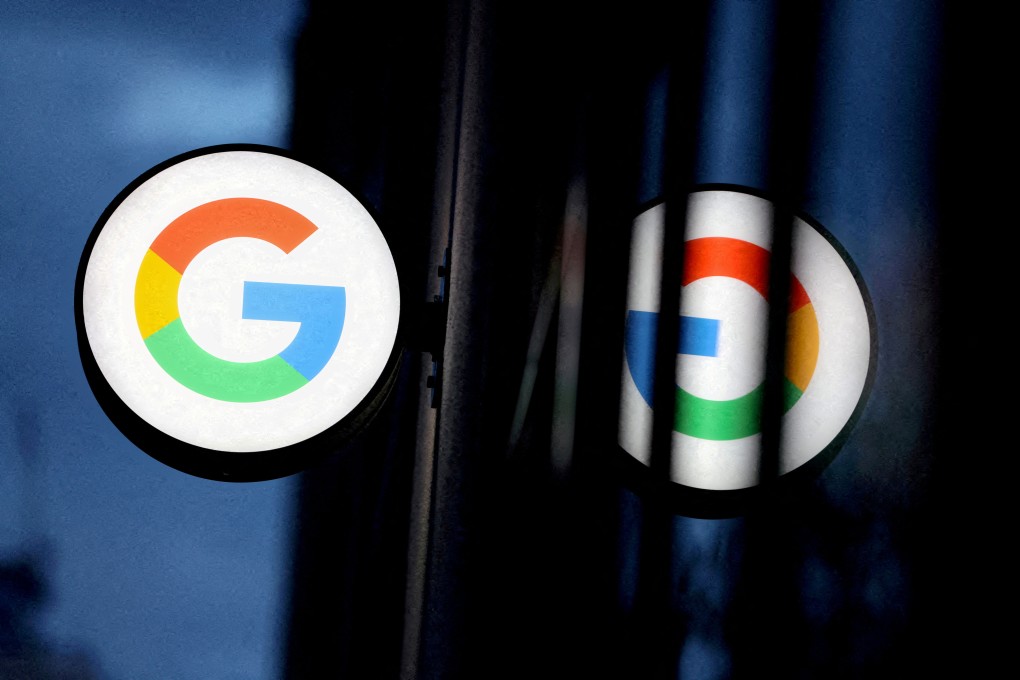
Alphabet’s Google released on Tuesday new details about the supercomputers it uses to train its artificial intelligence (AI) models, saying the systems are both faster and more power-efficient than comparable systems from Nvidia Corp.
Google has designed its own custom chip called the Tensor Processing Unit, or TPU. It uses those chips for more than 90 per cent of the company’s work on AI training, the process of feeding data through models to make them useful at tasks such as responding to queries with humanlike text or generating images.
The Google TPU is now in its fourth generation. Google on Tuesday published a scientific paper detailing how it has strung more than 4,000 of the chips together into a supercomputer using its own custom-developed optical switches to help connect individual machines.
Improving these connections has become a key point of competition among companies that build AI supercomputers because so-called large language models that power technologies like Google’s Bard or OpenAI’s ChatGPT have exploded in size, meaning they are far too large to store on a single chip.
The models must instead be split across thousands of chips, which must then work together for weeks or more to train the model. Google’s PaLM model – its largest publicly disclosed language model to date – was trained by splitting it across two of the 4,000-chip supercomputers over 50 days.
Google said its supercomputers make it easy to reconfigure connections between chips on the fly, helping avoid problems and tweak for performance gains.
“Circuit switching makes it easy to route around failed components,” Google Fellow Norm Jouppi and Google Distinguished Engineer David Patterson wrote in a blog post about the system. “This flexibility even allows us to change the topology of the supercomputer interconnect to accelerate the performance of an ML (machine learning) model.”